Because of its ease of implementation, computational efficiency and the fact that it generates multiple history-matched models, which conceptually allows one to characterize the uncertainty in reservoir description and future performance predictions, the ensemble Kalman filter (EnKF) provides a highly attractive technique for history matching production data. Despite these attractive features, EnKF had not been widely adopted as a standard history matching tool because of questions about its robustness and reliability. In particular, a relatively small ensemble is necessary for computational efficiency but limits the degrees of freedom to assimilate data, often results in a significant underestimation of variance, can introduce spurious long-distance correlations between elements of the state and data vectors, can result in filter divergence and a poor estimate of the conditional mean. Covariance localization represents the primary method for mitigating the negative effects of a small ensemble size. This research project focus on determining optimal covariance localization procedures for petroleum reservoir applications.
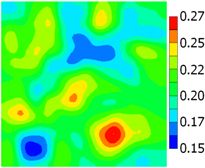
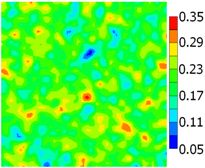
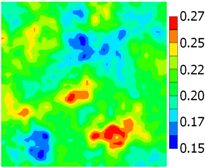
Example illustrating that covariance localization helps to improve the estimate of conditional mean for a linear problem.
Recent publications:
- Emerick, A.A. and Reynolds, A.C.: History Matching a Field Case Using the Ensemble Kalman Filter with Covariance Localization – SPE Reservoir Evaluation and Engineering, 2011.
- Emerick, A.A. and Reynolds, A.C.: Combining Sensitivities and Prior Information for Covariance Localization in the Ensemble Kalman Filter for Petroleum Reservoir Applications, Computational Geosciences, 2010.