The ensemble Kalman filter (EnKF) has recently become a popular history-matching tool largely because of its computational efficiency and ease of implementation. While EnKF has improved previous history matches obtained manually in several field cases, and often appears to give reasonable results for realistic synthetic history-matching problems, one cannot theoretically establish that EnKF samples correctly the posterior probability distribution for the reservoir model parameters or correctly assesses the uncertainty in the production forecast unless strong assumptions of Gaussianity and linearity apply. In multiphase flow problems, the relationship between data and reservoir model parameters and the primary simulation variables is highly nonlinear, so the theoretical justification for obtaining a correct assessment of the uncertainty in model parameters and future performance predictions does not hold. On the other hand, it is well known that the Markov chain Monte Carlo (MCMC) method sample correctly in the limit. However, its direct application to reservoir problems can be prohibitively expensive computationally. This research project focus on combining EnKF and MCMC for improved history matching and better characterization of uncertainty in reservoir simulation models.
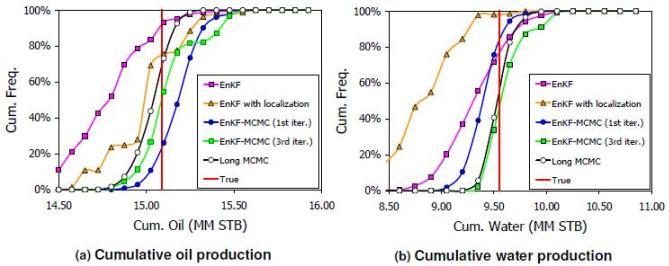
Cumulative distributions obtained from EnKF, EnKF with localization and EnKF-MCMC. The curves in black represent the reference distributions obtained from MCMC using a chain with 2 million proposals
Recent publications:
- Emerick, A.A. and Reynolds, A.C.: Combining the Ensemble Kalman Filter with Markov Chain Monte Carlo for Improved History Matching and Uncertainty Characterization – accepted for SPE Journal, 2011.
- Emerick, A.A. and Reynolds, A.C.: EnKF-MCMC – paper SPE 131375, SPE EUROPEC/EAGE Annual Conference and Exhibition, Barcelona, Spain, 14-17 June 2010.